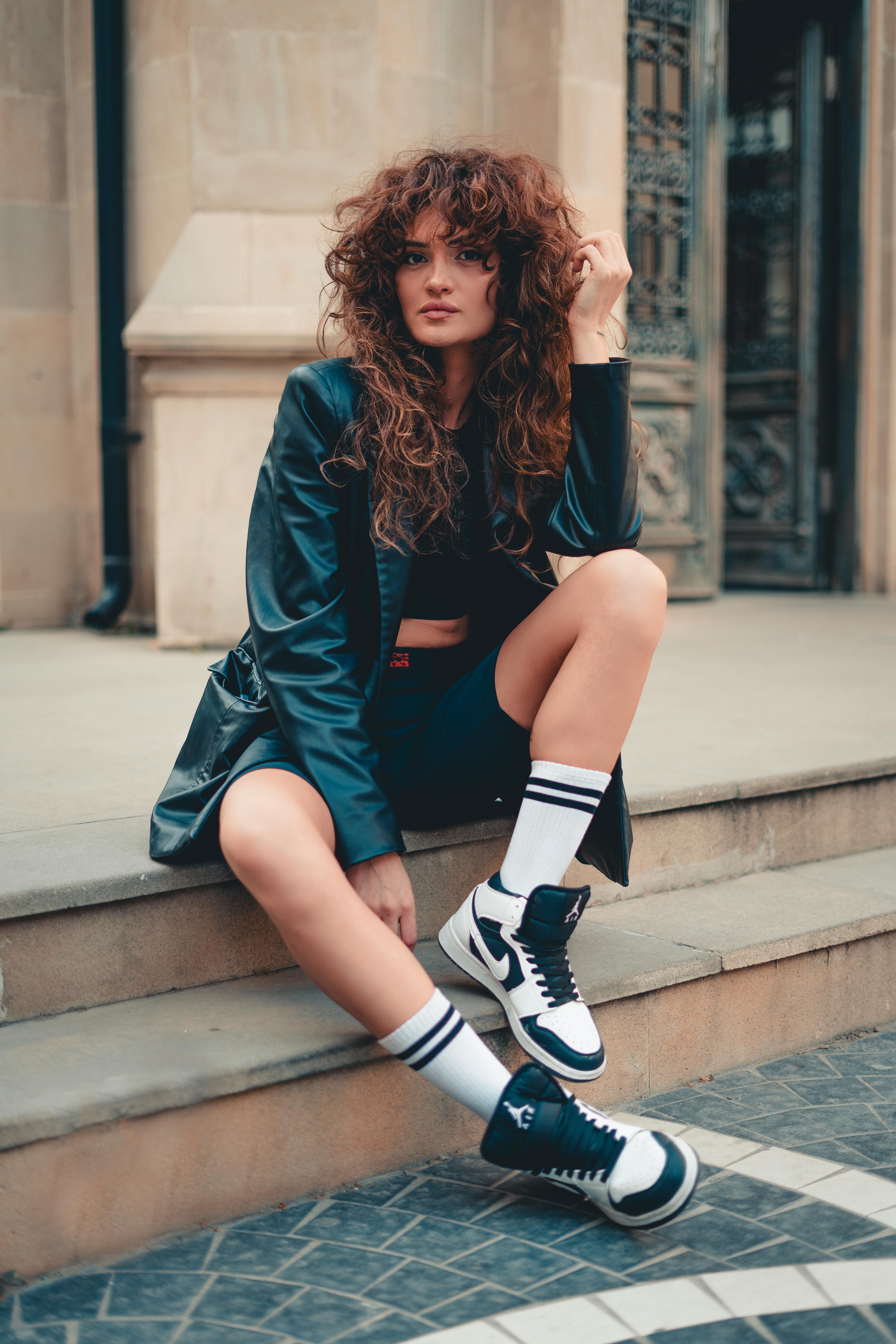
Effective Ways to Calculate IQR and Improve Data Analysis in 2025
Understanding Interquartile Range (IQR)
To fully grasp the concept of **interquartile range (IQR)**, one must appreciate its significance in **data analysis**. The IQR is a robust measurement of statistical dispersion, representing the middle 50% of data points in a statistical data set. Calculating the IQR helps analysts understand data variability and identify potential outliers. In 2025, it remains an essential element in **statistical analysis** as it provides valuable insights into data distribution without being affected by extreme values or skewness.
What is IQR?
The **IQR**, or **interquartile range**, is defined as the difference between the first quartile (Q1) and the third quartile (Q3) in a data set. Mathematically, it can be expressed using the **IQR formula**:
IQR = Q3 - Q1. This calculation allows researchers and statisticians to quantify the range within which the central majority of data points lie, effectively measuring statistical range and reducing the influence of outliers on critical data insights. Hence, mastering the IQR is pivotal for effective **comparative statistics**.
The Importance of IQR in Statistical Analysis
In **descriptive statistics**, the IQR plays a crucial role in helping to visualize data spread and understand underlying trends. Using methods like **box plot interpretation**, one can instantly assess data variability and detect outliers, thus making the IQR essential for accurate **data evaluation**. This reflects its importance in robust statistical methods as it not only aids in **finding quartiles** but also in making informed decision-making backed by solid data evidence.
IQR and Box Plots
One powerful application of the interquartile range is through **box plot analysis**. A box plot graphically illustrates the **quartile calculation**, providing a clear visual representation of the distribution and dispersal of the data. The central box encompasses the middle 50% of data (the IQR), while the "whiskers" extend to the smallest and largest values that are not outliers. Understanding how to visualize IQR using box plots is vital for presenting complex data insights ultimately facilitating effective storytelling through statistics. Such visual tools become indispensable in portraying the **spread of data** in a clear and concise manner.
Effective Methods to Calculate IQR
Calculating the IQR involves systematic steps to ensure accuracy. Below are effective methods that can be applied for proficient calculation:
Step-by-Step Guide to Calculating IQR
Calculating the **IQR** involves these steps:
1. **Arrange the data set** in ascending order.
2. **Determine the first** (Q1) and third (Q3) quartiles.
3. Subtract Q1 from Q3. This straightforward approach is crucial for ensuring consistent **data manipulation** and accurate representation of **variability measurement** in your analysis. You can refer to the visual representation below for clarity:
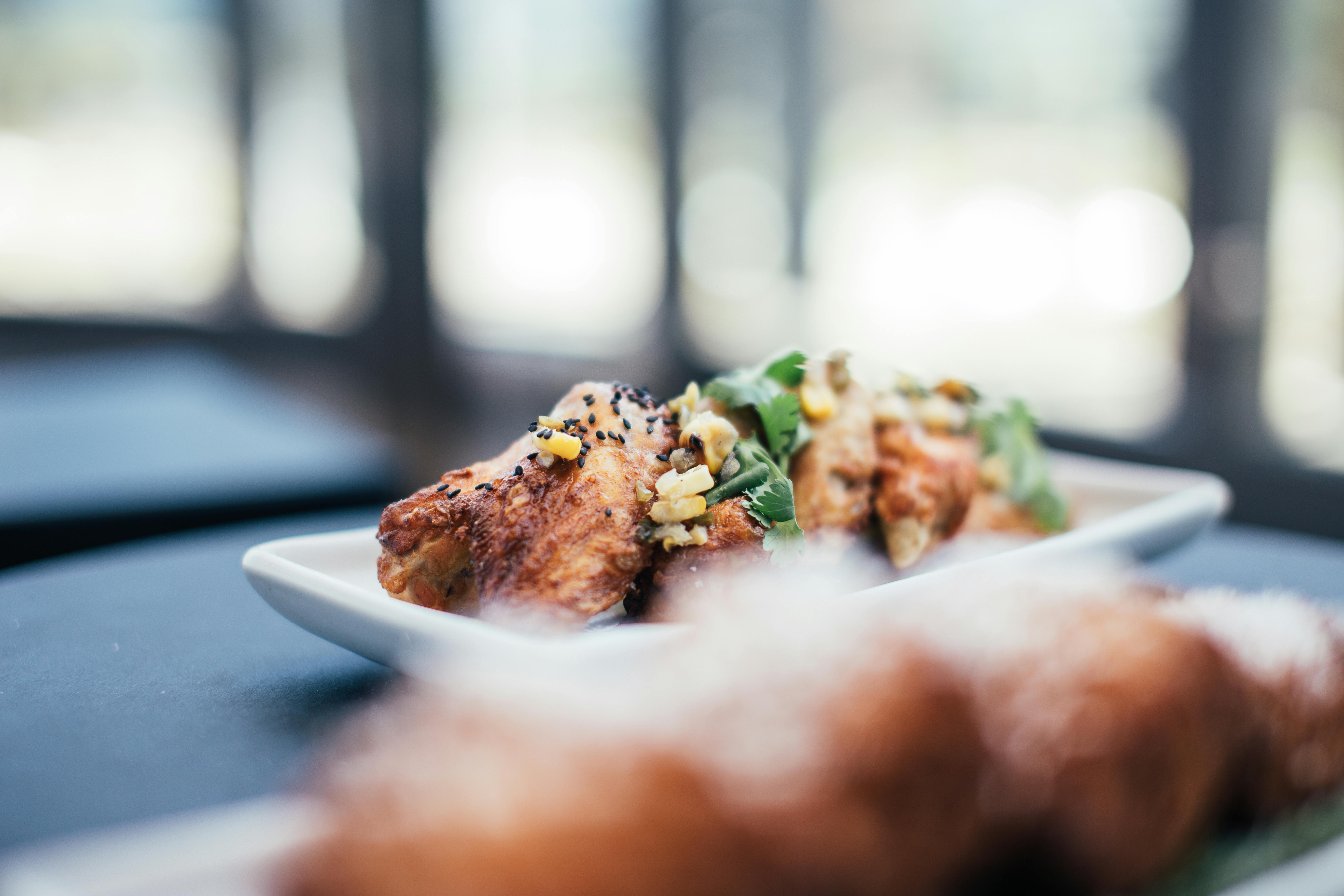
IQR Formula Examples
To master the **IQR formula**, let’s explore examples showcasing its calculation:
- Given the data set [4, 7, 1, 8, 10, 3, 5], after sorting to [1, 3, 4, 5, 7, 8, 10]:
- First Quartile (Q1) = 3
- Third Quartile (Q3) = 7
- Therefore, IQR = Q3 - Q1 = 7 - 3 = 4. This example exemplifies how consistent application of the **IQR method** yields essential insights, assisting in assessing data spread, making it clearer for analysts to understand the data relationship.
IQR Application in Data Analysis
The significance of the **IQR value** emerges prominently in various analytical scenarios, such as outlier detection. By identifying values that sit outside the calculated ranges, statisticians can pinpoint anomalies that may skew interpretation. This utility extends to **IQR outliers** detection, ensuring that data integrity is maintained throughout analysis. Recognizing these applications of the IQR allows for better understanding of **data statistics**, elucidating the relevance of quartiles in navigating distinct data landscapes.
Improving Data Insight with IQR
The interquartile range is not only significant for determining data spread but also plays a pivotal role in drawing insights and conclusions from statistical data distributions. Here’s how to leverage the IQR effectively:
IQR in Research Analysis
**Understanding IQR** within the context of research amplifies its relevance. In studies involving population samples or comparative assessments, the IQR serves as a vital indicator of data spread under varied conditions. Its principle remains concentrated on providing a clear depiction of deviations, always marrying together the concepts of **variability measurement** with illustrative statistics. Hence, it becomes a **statistical tool** that empowers researchers to infer patterns and predict outcomes based on existing data metrics.
Descriptive Statistics and IQR
In the arena of **descriptive analysis**, harnessing the power of the IQR leads to better-informed hypotheses and theories. For instance, when analyzing income levels across a population, calculating the IQR can uncover variations within socio-economic strata, which ignites further inquiry into the factors influencing these variations. Therefore, learning to interpret **IQR in data** responsibly is paramount for both novice and veteran statisticians, helping them appreciate the subtleties surrounding data interpretation.
IQR Solutions for Data Interpretation
Finally, implementing **statistical techniques** that employ the IQR allows for outlier detection and data refinement. By determining the range of normalcy within data, researchers can establish validation thresholds and discern meaningful anomalies versus noise. In this way, statistical insights achieved via the IQR enable competent **data analysis**, allowing practitioners to reshape their understanding of data-centric challenges while amplifying relevance in their interpretations. Understanding the IQR’s key role leads to enriched applicability across data endeavors.
Key Takeaways
- IQR is essential for understanding the dispersion of data sets and is resistant to outliers.
- Mastering the calculation steps allows for accurate data handling and facilitates robust analysis.
- IQR acts as a fundamental support tool in statistical techniques such as box plots.
- The application of IQR in research and descriptive statistics increases the value of data insights.
- Effective IQR implementation leads to improved data integrity and enhanced interpretations of variability.
FAQ
1. What is the significance of using the IQR in data analysis?
The **IQR significance** lies in its ability to summarize data spread effectively while minimizing the skewing impact of extreme values. This feature allows researchers to build cleaner statistical models and ensure that interpretations are reliable, ultimately promoting robust analytical results.
2. How can I visualize the IQR in data sets?
The IQR can be visualized using **box plot interpretation**. This graphical technique highlights the range, median, and potential outliers within a data set, allowing for immediate comprehension of data dispersion and facilitating insightful analysis.
3. What are common errors in finding IQR?
Errors often occur during the sorting process, miscalculating quartiles, or misrepresenting findings. Another common pitfall is not accounting for data sets with a low number of observations, which can disproportionately influence IQR results.
4. Can IQR be used in paired data analysis?
Yes, the **IQR relevance** extends to paired data, where it can flag variability across data points, highlighting significant differences in distributions. This ability renders it invaluable within research that examines datasets for comparative analyses.
5. What are some real-world examples where IQR is applied?
In fields such as finance, healthcare, and education, the **IQR application** facilitates identifying outlier transactions, patient health metrics, or student performance patterns. These insights are critical for making data-driven decisions that lead to improved outcomes within these sectors.