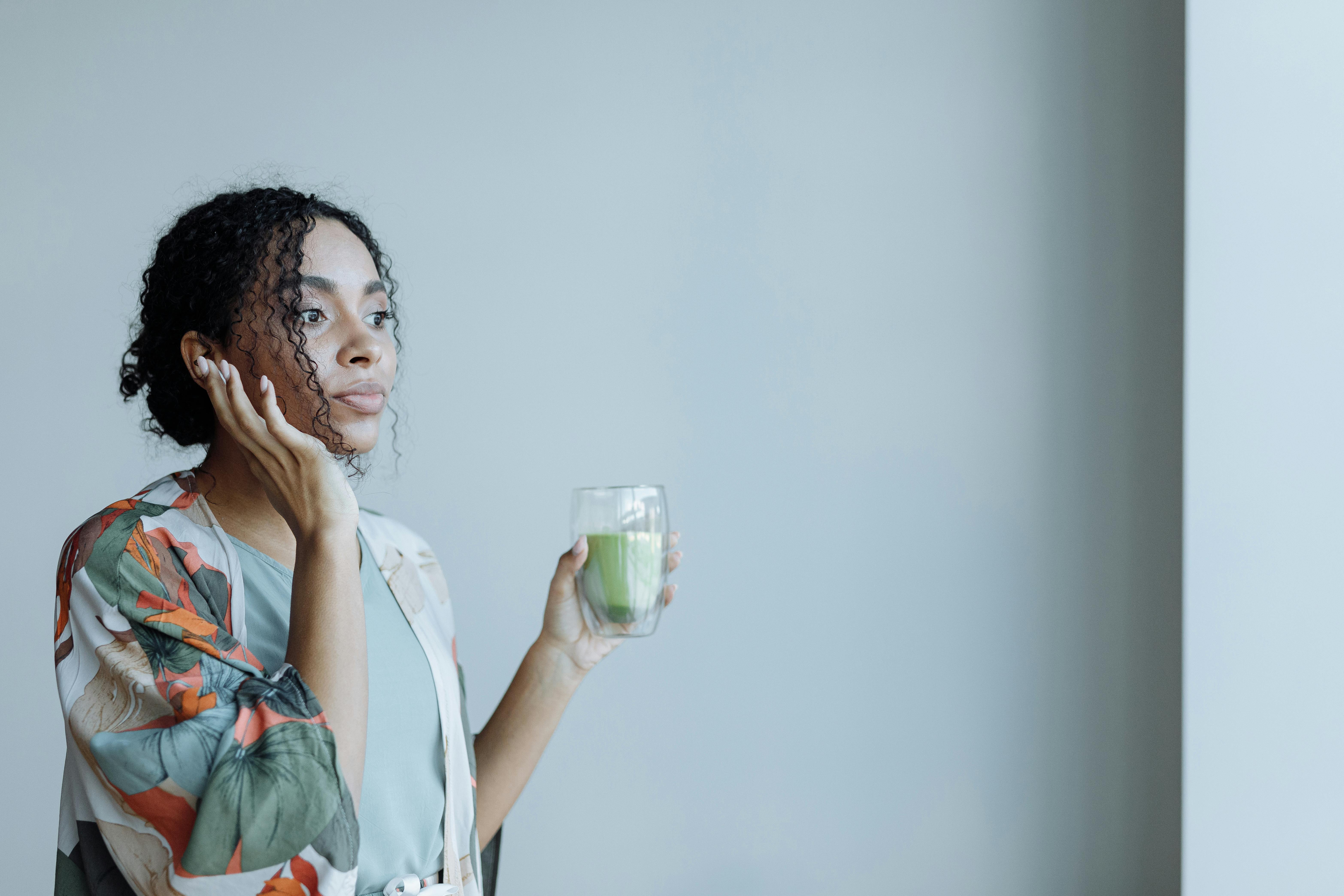
Essential Guide to Understanding and Calculating Uncertainty in 2025
The concept of uncertainty is a fundamental aspect of scientific research, measurements, and data analysis. In an era dominated by precision and accuracy, understanding how to calculate uncertainty has become increasingly important for researchers, engineers, and analysts alike. This guide focuses on the different methodologies for calculating uncertainty, examines various sources, and presents techniques for reducing measurement errors.
As our understanding of uncertainty evolves, so do the tools and techniques employed to quantify it. This article aims to provide a comprehensive overview of how to calculate uncertainty effectively, ranging from basic definitions to advanced statistical methods. Readers will also gain insights into uncertainty management and best practices for improving accuracy in scientific endeavors.
We will explore the various types of uncertainty, how they impact results, and the principles behind evaluating uncertainty. By delving into these topics, we prepare readers not only to calculate uncertainty accurately but also to improve their research reliability.
Key takeaways include understanding the uncertainty principle, mastering uncertainty calculation methods, and applying these techniques in real-world scenarios. In summary, this guide will empower you to navigate the intricate landscape of uncertainty in scientific measurement and research.
Understanding the Uncertainty Principle in Science
Building on the fundamentals of measurement, the uncertainty principle fundamentally roots itself in the nature of scientific inquiry. The uncertainty principle highlights the relationship between measurement accuracy and the inherent limitations in observing phenomena. It affects how scientists perceive and report their findings.
Definition of Uncertainty and Its Types
Uncertainty can be defined as the doubt or variance in a measurement or prediction. Acknowledging different uncertainty types is essential. These include systematic errors, which are predictable and often controllable, and random errors, which arise from unpredictable variations in measurement conditions.
Sources of Uncertainty in Measurements
The common sources of uncertainty include instrument precision, environmental factors, and human error. Understanding these factors allows researchers to better calibrate their instruments and methodologies, leading to improved measurement confidence.
The Role of Uncertainty in Scientific Research
In the context of scientific research, uncertainty plays a crucial role in the interpretation of data. For example, high levels of uncertainty may cast doubt on the significance of results, prompting the need for further analysis or experimentation. Thus, researchers must evaluate measurement accuracy carefully to draw valid conclusions.
Methods for Calculating Uncertainty in Measurements
With an understanding of the uncertainty principle established, we can delve into the various methodologies for uncertainty calculation. These methods enable researchers to quantify uncertainty systematically.
Statistical Tools for Uncertainty Analysis
Statistical tools are commonly employed for calculating measurement uncertainty. These tools include mean, standard deviation, and confidence intervals, which help quantify the expected variance in measurements. Furthermore, statistical methods such as Bayesian uncertainty analysis offer robust frameworks for managing uncertainty in a probabilistic context.
Error Propagation Techniques
Error propagation involves determining how measurement errors affect the overall uncertainty of calculations or derived results. Understanding how errors propagate through calculations is vital for estimating the final uncertainty linked to measurements accurately.
Calculating Uncertainty Using Monte Carlo Simulations
Monte Carlo simulations provide another effective method for uncertainty estimation. This technique uses random sampling to model complex systems and assess uncertainty under various scenarios, making it invaluable in predictive modeling.
Reducing and Managing Uncertainty in Experiments
Having explored various methods of calculating uncertainty, the next step emphasizes the significance of uncertainty management. Effectively reducing uncertainty can enhance measurement reliability and yield more accurate results.
Uncertainty Reduction Techniques
Uncertainty reduction techniques involve modifying experimental setups, improving instrument precision, and adopting standardized measurement protocols to enhance accuracy. Researchers must continually evaluate their methodologies for potential improvements.
Strategies for Managing Measurement Errors
Identifying potential errors early in the research process can mitigate their impact on results. By implementing strategies for managing uncertainty, such as systematic calibration processes and rigorous training for handling measurement instruments, researchers can significantly enhance result reliability.
Practical Examples of Uncertainty Management
Through real-world examples, researchers can observe how systematic monitoring and auditing of laboratory practices lead to enhanced precision and less variability. Case studies in fields like environmental assessment illustrate these principles in action, emphasizing the impact of proper risk management on uncertainty reduction.
Quantifying Uncertainty in Statistical Analysis
Transitioning to statistical perspectives, quantifying uncertainty plays a pivotal role in data interpretation. Understanding statistical significance amidst uncertainty is key to making informed conclusions in research.
Statistical Methods for Uncertainty Quantification
Statistical methods such as interval estimation and sensitivity analysis are crucial for evaluating uncertainty in statistical analysis. These methods allow researchers to assess the impacts of varying parameters on data interpretation.
Evaluating Uncertainty in Results
Effectively evaluating uncertainty in results not only bolsters credibility but also ensures that findings are reported in context. Tools like the Gaussian uncertainty model help quantify uncertainties across data points, providing insight into potential ranges of error.
Communicating Uncertainty Clearly
Communicating uncertainty is essential for educating audiences on the limitations of outcomes. Employing clear language and visual indicators such as error bars or confidence intervals can enhance understanding and promote transparency in research.
Conclusion: The Future of Uncertainty Calculations
As we progress towards more advanced scientific methodologies, understanding the nuances of uncertainty will be critical. This framework will guide future research endeavors, ensuring that measurements are not only accurate but transparently communicated.
By mastering uncertainty calculations, researchers can enhance the reliability of their findings. In conclusion, addressing uncertainty with systematic methodologies will lead to improved research outcomes across various scientific disciplines.